Computational Materials Modeling Database
The Computational Materials Modeling Database provides an integrated resource site of Virginia Tech researchers engaged in computational modeling of materials.
The purpose of the database is to promote partnerships between VT faculty, students, funding agencies, and external partners in areas of computational strength at Virginia Tech. We have divided the database by topical areas and invite you to explore the faculty pages included.
Research Areas
Computational Materials Modeling, Virginia Tech
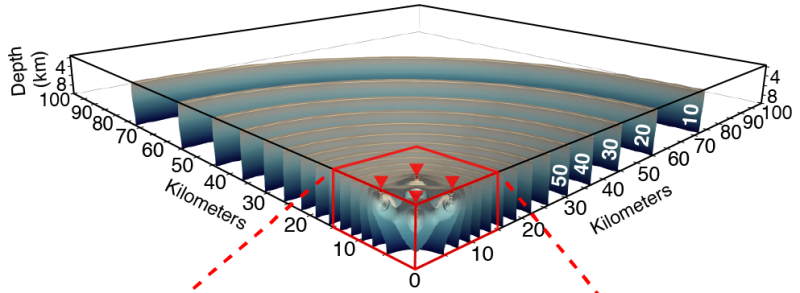
Fluid Mechanics
The study of fluid flow and mass transport in different media. Such as prediction of wind gusts in a hurricane, air flow over airplane wings, mass transport during manufacturing processes, molten material flow during casting, drug flow in patients, and blood flow in arteries and hearts.
Computational Materials Modeling, Virginia Tech
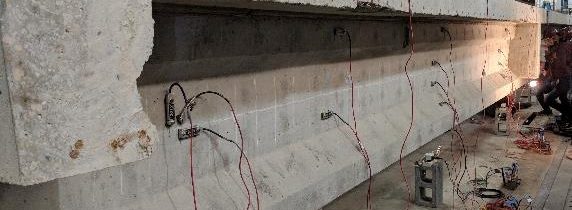
Fracture, Damage, and Impact Mechanics
The science- and physics-based computational models of failure criteria, crack initiation, propagation, and fatigue damage under different static or dynamic loads to revolutionize component designs.
Machine Learning, Computational Materials Modeling, Virginia Tech
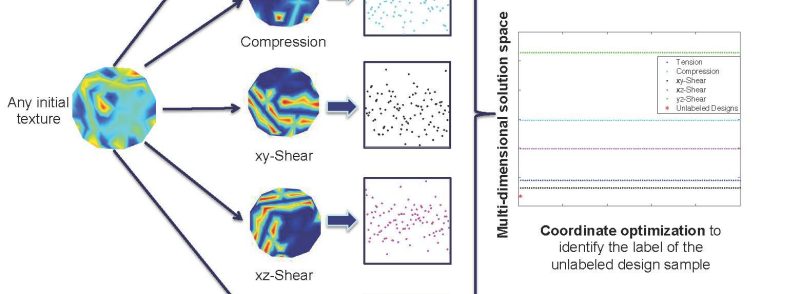
Machine Learning
Application of machine learning to understand and predict the behavior of materials, the use of algorithms and statistical models to effectively perform a simulation without using explicit theoretical physical models, relying on patterns and inference instead.
Multiphysics and Multiscale Modeling, Computational Materials Modeling, Virginia Tech
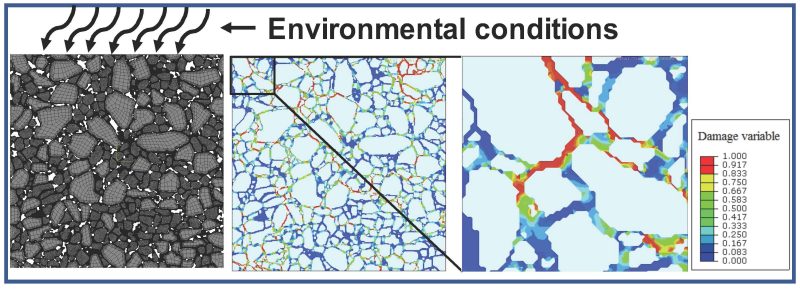
Multiphysics and Multiscale Modeling
Multi-physics and Multiscale modeling involve studying coupling among mechanical, thermal, electrical, magnetic, chemical, and moisture-absorption induced loads/effects on materials and structures across different length and time scales.
Computational Materials Modeling, Virginia Tech
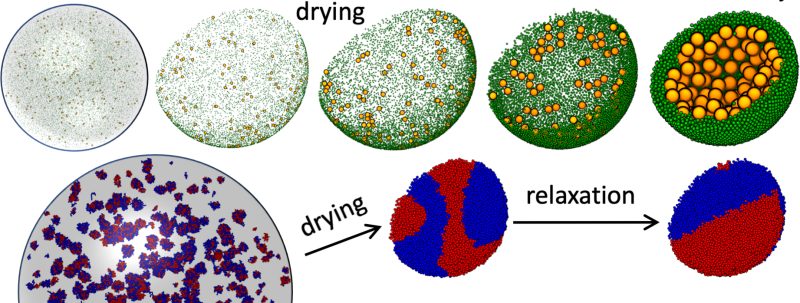
Soft Matter
Soft matter is the study of materials of which the physical behavior is controlled by an energy scale comparable to room temperature thermal energy. These materials include polymers, colloids, liquid crystals, foams, gels, granular matter, and biological materials.
Uncertainty Quantification and Data-Driven Modeling, Computational Materials Modeling, Virginia Tech

Uncertainty Quantification and Data-Driven Modeling
Uncertainty quantification uses statistical principles to quantify the probability of a designed part successfully functioning during its anticipated life span, and/or the accuracy of test results. Data-driven modeling tries to learn from available data instead of traditional modeling with hard-coded instructions.